Exploring AMD’s Ambitious ROCm Initiative
Machine Learning and AI
ROCm is built to accommodate future technologies, and the future is machine learning and artificial intelligence. Many of the high-performance computer systems that depend on AMD’s GPU-based computing environment are used with AI research and development, and ROCm is designed from the ground up as a versatile and complete platform for machine learning and AI (Figure 3).
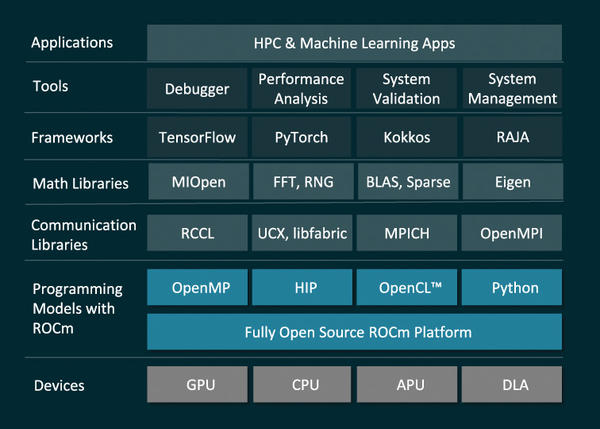
Recent versions of the TensorFlow and PyTorch machine-learning frameworks provide native ROCm support. ROCm also supports the MIOpen machine-learning library [8]. MIOpen serves as a middleware layer between AI frameworks and the ROCm platform, supporting both OpenCL and HIP-based programming environments. The latest version of MIOpen includes optimizations of new workloads for Recurrent Neural Networks and Reinforcement Learning, as well as Convolution Neural Network acceleration.
The latest version of ROCm adds enhanced support for distributed training and the deep learning model. Many example codes have already been validated on AMD Radeon Instinct GPUs for TensorFlow on ROCm.
Containers and ROCm
A recent trend in HPC is increased reliance on containers. Containers are easy to extend and adapt to specific situations, and a containerized solution is an efficient option for many HPC configurations and workloads. The ROCm developers have continued to improve and expand ROCm support for container technologies, such as Docker and Singularity.
The latest version of ROCm includes support for Kubernetes, Slurm, OpenShift, and other important tools for managing and deploying container environments. Also, the ROCm-docker repository contains a framework for building the ROCm software layers into portable Docker container images. If you work within a containerized environment, ROCm’s Docker tools will allow you to integrate ROCm easily into your existing organizational structure.