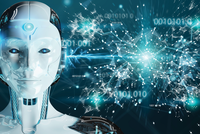
Lead Image © sebastien decoret, 123RF.com
The limits and opportunities of artificial intelligence
Rocket Science
Despite, or perhaps because of, research successes in artificial intelligence (AI), powerful AI seems to be further away today than it was 10 years ago. Will AI ever step toward automated super-intelligence, or are artificial narrow-band geniuses all that can be expected for the foreseeable future?
ADMIN interviewed Peter Protzel, who studied Electrical Engineering and received his Ph.D. from the University of Braunschweig, Germany, in 1987. He then spent five years as a staff scientist at the NASA Langley Research Center in Virginia, followed by seven years at the Bavarian Research Center for Knowledge-Based Systems in Erlangen, Germany, where he headed the neural networks research group. Since 1998 he has been a full professor for automation technology at the University of Chemnitz, Germany.
ADMIN: Prominent advocates of the idea of super-intelligence – Ray Kurzweil, Stephen Hawking, Elon Musk – are apparently afraid of its independence. Our current experience, on the other hand, is an AI that distinguishes dog from cat when trained but fails at the simplest tasks if not prepared for them. How does that add up?
Peter Protzel: The core of the problem in the AI discussion is a clean distinction of terms. We always need to make a strict distinction between whether we are talking about the current "narrow" or "weak" AI or about the "general" or "strong" AI imaginable in the future (artificial general intelligence, AGI). The terms "narrow" and "general" illustrate the difference: The narrow AI we have now is a machine that we construct (and train) to solve a specific problem. A facial recognition system does not recognize speech or translate text. AlphaGo plays Go, but not Nine Men's Morris.
The interesting thing about narrow-band AI is that in deep learning, for example, we now have a method that solves quite
...Buy this article as PDF
(incl. VAT)
Buy ADMIN Magazine
Subscribe to our ADMIN Newsletters
Subscribe to our Linux Newsletters
Find Linux and Open Source Jobs
Most Popular
Support Our Work
ADMIN content is made possible with support from readers like you. Please consider contributing when you've found an article to be beneficial.
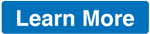