« Previous 1 2
Kick-start your AI projects with Kubeflow
Quick Start
Starting EKS
Because Kubeflow is based on Kubernetes, a working EKS cluster is a mandatory requirement for Kubeflow on AWS. I recommend the eksctl
command-line tool, which communicates directly with the AWS EKS API and creates the required cluster in just a few minutes. The following example assumes that all commands are run on Ubuntu 22.04, but most of the commands will probably work on other distributions with just a few minor changes. To use eksctl
on Ubuntu, first install the required binary directly from Amazon with the commands in Listing 1.
Listing 1
Installing eksctl and AWS
$ ARCH=amd64 $ PLATFORM=$(uname -s)_$ARCH $ curl -sLO "https://github.com/eksctl-io/eksctl/releases/latest/download/eksctl_$PLATFORM.tar.gz" $ curl "https://awscli.amazonaws.com/awscli-exe-linux-x86_64.zip" -o "awscliv2.zip" $ tar -xzf "eksctl_$PLATFORM.tar.gz" -C /tmp && rm "eksctl_$PLATFORM.tar.gz" $ unzip -u awscliv2.zip $ sudo mv /tmp/eksctl /usr/local/bin $ sudo ./aws/install
A call to eksctl --version
should then display the version information of the tool on the console. Although eksctl
is now basically ready for use, it lacks the access credentials for AWS. You can store these with the aws configure
command. AWS prompts for the Access Key ID and the Secret Access Key, two pieces of information that can be found directly on the overview page of your AWS account. Depending on your personal preferences, you can also specify a default region and a default output format, but it is not mandatory. Once AWS and eksctl
are ready to go, the next step is to create an EKS cluster (Listing 2).
Listing 2
Creating an EKS Cluster
export AWS_ACCOUNT=<Access Key ID> export CLUSTER_REGION=eu-central-1 export CLUSTER_NAME=kubeflow-1 export PROFILE_NAME=kubeflow-user export PROFILE_CONTROLLER_POLICY_NAME=kubeflow-user eksctl create cluster --name ${CLUSTER_NAME} --version 1.25 --region ${CLUSTER_REGION} --nodegroup-name linux-nodes --node-type <m5.xlarge> --nodes 5 --nodes-min 5 --nodes-max 10 --managed --with-oidc eksctl create iamserviceaccount --region ${CLUSTER_REGION} --name ebs-csi-controller-sa --namespace kube-system --cluster ${CLUSTER_NAME} --attach-policy-arn arn:aws:iam::aws:policy/service-role/AmazonEBSCSIDriverPolicy --approve --role-only --role-name AmazonEKS_EBS_CSI_DriverRole eksctl create addon --name aws-ebs-csi-driver --cluster ${CLUSTER_NAME} --service-account-role-arn arn:aws:iam::$(aws sts get-caller-identity --query Account --output text):role/AmazonEKS_EBS_CSI_DriverRole --force
Some of the details in the command shown here are variable; you can or need to adjust them – in particular, the value for AWS_ACCOUNT
, which determines which of the stored sets of access credentials eksctl
uses. The parameter for --node-type
can also be changed. The advice, though, is only to choose a smaller instance size if this is a test cluster. In contrast, production systems will possibly need an even larger instance type depending on the workload, although keeping a watchful eye on the invoice amounts in AWS is definitely recommended.
Once you have completed this step, the next step is to create the Kubeflow cluster by first preparing the folder with the scripts and integration components for AWS from the Kubeflow Git directory and navigating to a subfolder of the freshly checked-out source code:
$ git clone https://github.com/awslabs/kubeflow-manifests/ $ cd kubeflow-manifests/tests/e2e/utils/cognito_bootstrap/ [... edit config.yaml ...]
Of particular interest is the file config.yaml
, although it is largely empty by default. You need to modify several values in this file: hostedZoneId
and name
below the route53.rootDomain
element and cluster.name
, cluster.region
, and name
below the cognitoUserpool
element. The last value is variable, but a mnemonic name is recommended. Note that the names that follow cluster.name
and cluster.region
must match the values you used when creating the EKS cluster.
Finally, change the value of name
below the route53.subDomain
element to define the subdomain in which the Kubeflow API will later be accessible, which is why it must be a subdomain of the main domain stored in Route 53 (e.g., true-west.cloud
and kubeflow.true-west.cloud
in this example).
Finally, move up two directory levels and launch the integration script:
$ cd kubeflow-manifests/tests/e2e/ $ PYTHONPATH=.. python utils/cognito_bootstrap/cognito_pre_deployment.py [... Pre-deployment run ...]
The script automatically creates resources in AWS Route 53 and AWS Cognito that Kubeflow will later need to create a functional load balancer and integrate with Cognito. The script also automatically creates the subdomain you need in Route 53 and extends the config.yaml
file (which you edited manually previously) so that it contains valid values at this point in time. After the pre-deployment step, you will later have – as the experienced administrator will already have guessed – a post-deployment step.
Please note that depending on the time of day and the instances used in AWS, both creating the EKS cluster and handling the required preparations can take 20 minutes or longer. However, unless the tools explicitly display an error message, you do not need to worry; AWS is just a little slow at times. Once this preparatory step has been completed, continue with the Kubeflow deployment.
Creating Namespaces
A minor adjustment still needs to be made because of the way in which Kubeflow implements a form of multiclient capability internally and how the integration component is implemented in Cognito.
Under the hood, Kubeflow is based on the idea of namespaces. The term is probably familiar to people from the Kubernetes universe, because namespaces also exist there. A Kubernetes namespace is always part of a Kubeflow namespace, as well, but the Kubeflow namespace also includes a user configuration and a set of rules that grant the newly created Cognito user access to a Kubeflow namespace.
Kubeflow creates a namespace for a user by default if the user does not have one when they first log in. This function is disabled in the default Kubeflow configuration, though. After a setup without any customization, users could therefore log into Kubeflow but not work with it. To change this behavior, change to the subdirectory in the kubeflow-manifests/
folder,
$ cd kubeflow-manifests/charts/apps/central-dashboard/templates/ConfigMap/
and change the value of CD_REGISTRATION_FLOW
to true
in the centraldashboard-parameters-kubeflow-ConfigMap.yaml
file. This adjustment is followed by the Kubeflow deployment:
$ cd kubeflow-manifests/ $ make deploy-kubeflow INSTALLATION_OPTION=kustomize DEPLOYMENT_OPTION=cognito
Again, you will need to be patient: More than 70 different services and well over 100 containers need to be downloaded and started in a process that usually takes 15 minutes or more. The wait is worthwhile: Kubeflow will basically be rolled out and ready for use. Of course, you can't access it as yet because the Cognito configuration and the associated load balancer are missing.
The last step of the process is to create both of these elements to conclude the setup:
$ cd kubeflow-manifests/tests/e2e/ $ PYTHONPATH=.. python utils/cognito_bootstrap/cognito_post_deployment.py
The Kubeflow dashboard is now available for login on kubeflow.<subdomain> (e.g., https://kubeflow.k8s.true-west.cloud here). Now all that is missing is the user account in AWS Cognito, and you can create this in the normal way from the AWS GUI.
Unlike a DIY Kubeflow (e.g., on the basis of deployKF), all the components will work smoothly immediately after completing the setup in the EKS-based variant. Pipelines can be created and managed, just like new notebooks or server applications to serve (trained) models for sharing with the outside world. Kubeflow even comes with some example pipelines out of the box.
It is beyond the scope of this article to go into detail about how Kubeflow works and how models can be created and trained in it, but some sample projects and comprehensive instructions can be found online.
Cleanup
Cleaning up after working with Kubeflow does not involve anything like the number of hoops you had to jump through to install the environment. All it takes is:
$ kubectl get svc --all-namespaces $ kubectl delete svc <service> $ eksctl delete cluster --name ${CLUSTER_NAME}
The first command displays all the services stored in the cluster. kubectl
works smoothly after creating a cluster with eksctl
, because eksctl
stores the access data locally for each newly created cluster and sets up environment variables to ensure that the right credentials are used.
The last two lines delete services you no longer need and clear the cluster again when done, provided it was set up as described in the example. If the delete
command returns the value 0
, the cluster has been removed successfully and is no longer using any resources that would add to your bill (Figure 5).
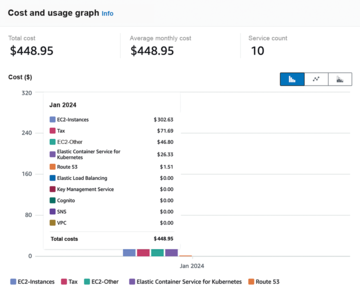
According to the process described, a Kubeflow installation can be set up from scratch at any time. The important thing is to reset config.yaml
for Cognito before the pre-deployment step so that it only contains the information you need for the domain you will be using. The deployment tools modify the file several times during setup. After completing the Kubeflow deployment, it can no longer be used to set up another new cluster.
Infos
- Kubeflow: https://www.kubeflow.org
- Kubeflow-AWS Cognito guide: https://awslabs.github.io/kubeflow-manifests/docs/deployment/cognito/
« Previous 1 2
Buy this article as PDF
(incl. VAT)
Buy ADMIN Magazine
Subscribe to our ADMIN Newsletters
Subscribe to our Linux Newsletters
Find Linux and Open Source Jobs
Most Popular
Support Our Work
ADMIN content is made possible with support from readers like you. Please consider contributing when you've found an article to be beneficial.
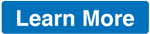