« Previous 1 2 3 Next »
Tool your HPC systems for data analytics
Get a Grip
Interactivity
A key aspect of data analytics is interacting with the analysis itself. Interactivity can mean multiple things. One form involves manual (i.e., interactive) preparation of the data for analysis, such as examining the data for outliers.
An outlier can be one data point or several data points that lie outside the expected range of data. A more mathematical way of stating this is that an outlier is an observation that is distant from other observations (you can substitute the word "measurement" for "observation"). The sources of outliers vary and include experimental error, instrument error, and human error. Typically, either the use of robust analysis methods that can tolerate outliers or removal of outliers from the data is desirable. The process of removing outliers is something of an art that requires scrutinizing the data, often with the use of visual analysis methods.
Sometimes, outliers are retained in the data set, which requires that you use a new or different set of tools. A simple example uses the idea that the median of a data set is more robust [27] than the mean (average). If you take a value in a data set and make it extremely large (approaching infinity), then the mean obviously changes. However, the median value changes very little. This doesn't mean the median is necessarily a better statistic than the mean, just that it is more robust in the presence of outliers. It may require several different analyses of the data set to understand the effect of outliers on the analysis. Robust computations might have to be employed if the presence of outliers greatly affects non-robust analysis. In either case, it takes a fair amount of analysis either to find the outliers or to determine what analysis techniques are most appropriate.
Visualization is a key component in data analytics. The human mind is very good at finding patterns, especially in visual information, so researchers plot various aspects of data to get a visual representation that can lead to some sort of understanding or knowledge. During data analysis, the plots used are not always the same, because the plots need to adapt to the data itself. Researchers might want to try several kinds of charts on a single data set to better understand the data. Unfortunately, it is difficult to know which charts use a priori . Whatever the case, DA systems need compute nodes with graphics cards.
HPC systems are not typically equipped with graphics cards for visualizing data; they are typically used for computation, with the results pulled back either to an interactive system or a user's workstation. Some HPC systems offer what is termed "remote visualization." The University of Texas Advanced Computing Center (TACC) has a system named Longhorn [28] that was designed to provide remote visualization and computation in one system. HPC systems like this allow researchers to get an immediate view of their work, so they can either make changes to the computations or change the analysis.
Data analytics is not like a typical HPC application that is run in batch mode with no user interaction. On the contrary, data analytics may require a great deal of interactivity.
Data Analytics Pipeline
Data analytics is a young and growing field that is trying to mature rapidly. As a result, many computations might be needed to arrive at some final information or answers. To get there, the computations are typically done in what is called a "pipeline" (also called a workflow). The phrase is commonly used in biological sequence analysis referring to a series of sequential steps taken to arrive at an answer. The output from one step is the input to the next step. Data analytics is headed rapidly in this same direction, but perhaps without explicitly stating it. Data analytics starts with raw data and then massages and manipulates it to get it ready for analysis. The analysis typically consists of a series of computational steps to arrive at an answer. Sounds like a pipeline to me.
Some of the steps in a DA pipeline might require visual output, others an interactive capability, or you might need both. Each step may require a different language or tool, as well. The consequence is that data analytics could be very complex from a job flow perspective. This can have a definite effect on how you design HPC systems and how you set up the system for users.
These characteristics – new languages, single-node runs (but lots of them), interactivity, and DA pipelines – are common to the majority of data analytics. Keep these concepts in mind when you deal with DA workloads.
Lots of Rapidly Changing Tools
Two features of DA tools is that they vary widely and change rapidly. A number of these tools are Java based, so HPC admins will have to contend with finding a good Java runtime environment for Linux. Moreover, given the fairly recent security concerns, you might have to patch Java fairly frequently.
Other language tools experience fairly rapid changes, as well. For example, the current two streams of Python are the 2.x and the 3.x series. Python 3.0 chose to drop certain features from Python 2.x and add new features. Some toolkits still work with Python 2.x and some work with Python 3.x. Some even work with both, although the code is a bit different for each. As an HPC admin, you will have to have several different versions of tools available on every node for DA users. (Hint: You should start thinking about using Environment Modules [29] or Lmod [30] to your advantage.)
One general class of tools that changes fairly rapidly is NoSQL databases. As the name implies, NoSQL databases don't follow SQL design guidelines and do things differently. For example, they can store data differently to adapt to a type of data and a type of analysis. They also forgo consistency in favor of availability and partition tolerance. The focus of NoSQL databases is on simplicity of design, horizontal scaling, and finer control over availability.
A large number of NoSQL databases are available with different characteristics (Table 5). Depending on the type of data analytics being performed, several of the databases can be running at the same time. As an administrator, you need to define how these databases are architected. For example, do you create dedicated nodes to store the database, or do you store the database on a variety of nodes that are then allocated by the resource manager to a user who needs the resource? If so, then you need to define these nodes with special properties for the resource manager.
Table 5
Databases
Name | URL |
---|---|
Wide column stores | |
HBase | http://hbase.apache.org/ |
Hypertable | http://hypertable.org/ |
Document stores | |
MongoDB | http://www.mongodb.org/ |
Elasticsearch | http://www.elasticsearch.org/ |
CouchDB | http://couchdb.apache.org/ |
Key value stores | |
DynamoDB | http://aws.amazon.com/dynamodb/ |
Riak | http://basho.com/riak/ |
Berkeley DB | http://en.wikipedia.org/wiki/Berkeley_DB |
Oracle NoSQL | http://www.oracle.com/technetwork/database/database-technologies/nosqldb/overview/index.html |
MemcacheDB | http://memcachedb.org/ |
PickleDB | https://pythonhosted.org/pickleDB/ |
OpenLDAP | http://www.openldap.org/ |
Graph | |
HyperGraphDB | http://www.hypergraphdb.org/index |
GraphBase | http://graphbase.net/ |
Bigdata | http://www.systap.com/ |
AllegroGraph | http://franz.com/agraph/ |
Scientific | |
SciDB | http://www.scidb.org/ |
« Previous 1 2 3 Next »
Buy this article as PDF
(incl. VAT)
Buy ADMIN Magazine
Subscribe to our ADMIN Newsletters
Subscribe to our Linux Newsletters
Find Linux and Open Source Jobs
Most Popular
Support Our Work
ADMIN content is made possible with support from readers like you. Please consider contributing when you've found an article to be beneficial.
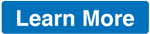